A quantitative understanding of evolution rests on the analysis of the mutation accumulation process in biological populations, but is largely limited to high-frequency mutations due to the resolution of conventional sequencing technologies. Based on the CirSeq technique developed by our collaborators from Prof. Raul Andino’s lab at UCSF that potentiates the faithful recovery of low-abundance mutations, the accumulation of mutations throughout the entire course of 37 serial passaged poliovirus populations were examined. Extremely rich mutation trajectory patterns were observed from the data.
Motivated by the observation, we have developed a set of mathematical models to describe the possible evolutionary trajectories of individual mutations, and a model selection procedure to determine the optimal interaction model for an individual mutational trajectory. These interaction models can then be used to infer the impact of evolutionary factors, including first-order factors such as mutation rate and the fitness cost of an independent mutation, as well as higher-order factors like genetic epistasis, clonal interference and genetic hitchhiking. We identify mutations consistent with a locus-independent behavior, which only depend on the first-order factors. Neutral mutations accumulate linearly, beneficial mutations grow exponentially before saturating and asymptotically reaching fixation and detrimental mutations accumulate until reaching mutation-selection balance. We also identify other types of mutations deviating from that simple model by the higher-order factors. For example, pairwise strong epistasis between beneficial and detrimental mutations lead to trajectory shifting of the detrimental mutation from the mutation-selection balance that it is expected to reach in the case of no mutational interaction to a higher balance level.
For mutations having a trajectory that allows for assessment by our models, we could describe the outline the fitness landscape. Clonal interference, followed by hitchhiking, appear to be the most prevalent interactions in the virus population. Epistasis, while presents, but does not significantly affect the distribution of mutational fitness on the short time scale examined in our study. Our study provides a comprehensive analysis of the allelic composition and how mutation rate, fitness, epistasis, clonal interference and hitchhiking influence population dynamics and evolution.
This research was submitted to bioRxiv at Jan 2020 (doi: https://doi.org/10.1101/2020.01.16.908129). Dr. Chang Chang (was under the supervision of Prof. Chao Tang, PKU), Dr. Simone Bianco (IBM Research - Almaden), and Dr. Ashley Acevedo (was under the supervision of Prof. Raul Andino, UCSF) contribute equally to this paper. The current affiliation of Dr. Chang Chang is Department of Molecular and Cellular Biology, Harvard John A. Paulson School of Engineering and Applied Sciences, Harvard University.
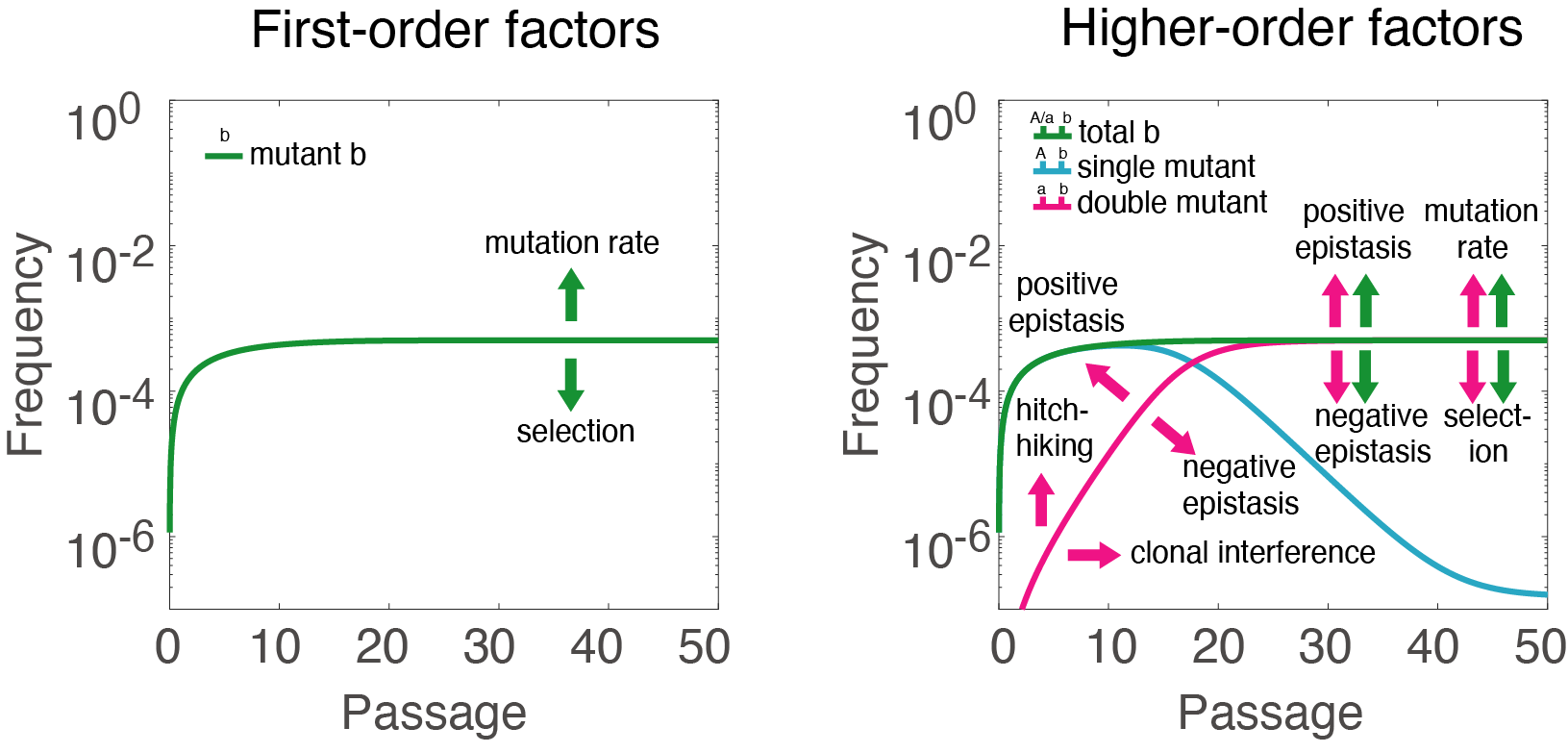