Cell segmentation always gets the biological investigators, who are sitting in front of the microscopicimages, head-scratching. It’s challenging because different labs meet different problems. Current segmentation methods relying on single boundary features are hardly transferred between labs, or even shared within the same lab. Here we provide a customizable and generalizable cell segmentation program - Cellbow.
Cellbow is a neural-network-based algorithm. Its structure is fixed, whereas the parameters are flexible-it can be automatically trained from the training dataset. For example, Cellbow-Fluo broadly segments fluorescent images of diverse cell types with no further training needed. Cellbow-BF robustly overcomes the inhomogeneous foci in budding and fission yeast cells imaging. Last but not the least, for a customized Cellbow, only a small set of sample images from the user may be needed for training.
To facilitate the application of Cellbow, we provide a website on which one can online test the software, as well as an ImageJ plugin for the user to visualize the performance before software installation. The tutorial can be easily found on the website: http://cls.pku.edu.cn:808/online/home/.
This work is published in Quantitative Biology volume 8, pages, 245–255(doi: https://www.biorxiv.org/lookup/doi/10.1101/2020.04.21.052597).CQB graduate students Huixia Ren and Mengdi Zhao contributed mostly to this work.
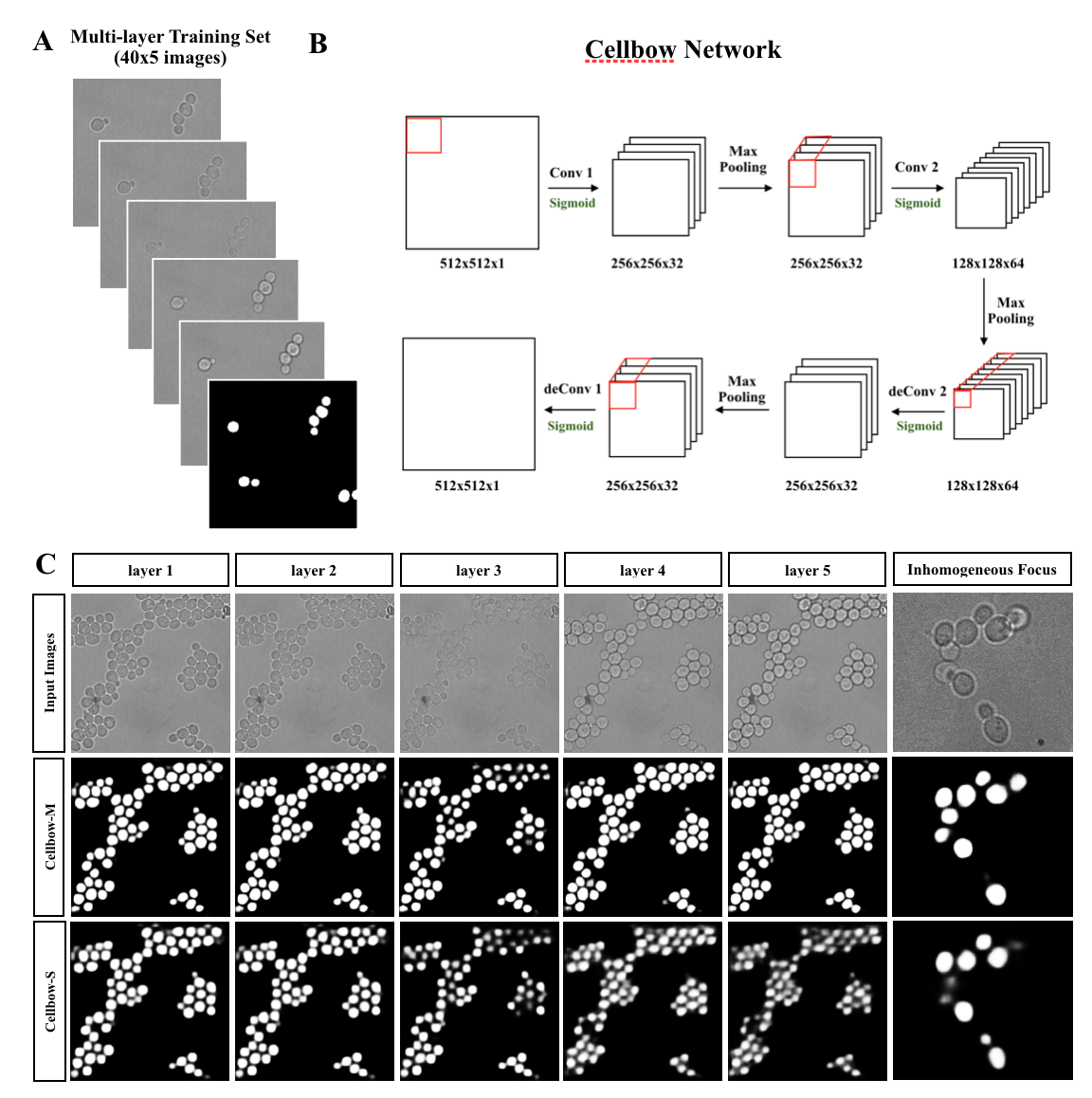
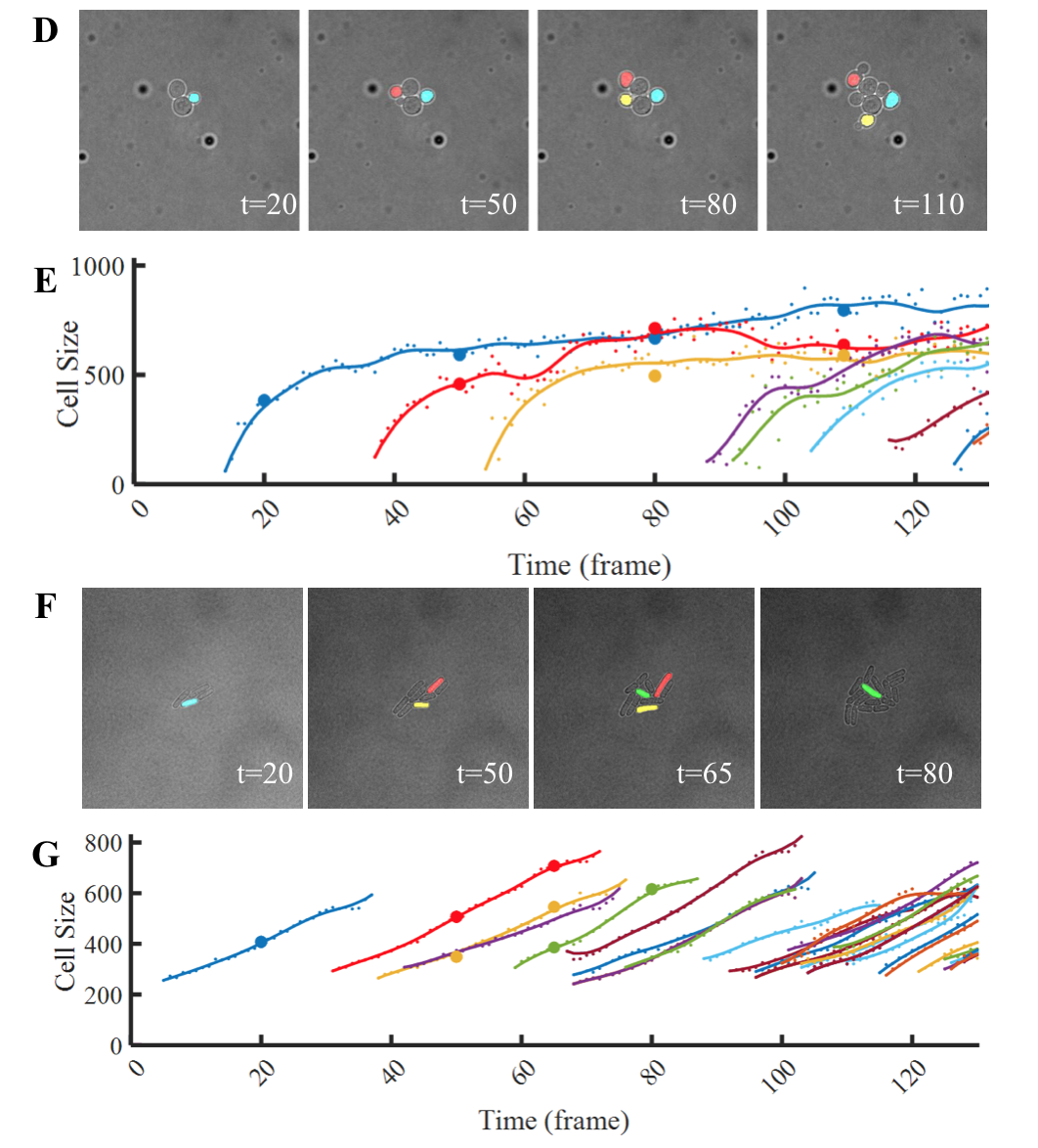