Research interests
Our research focuses on cancer immunology and immunotherapy and aims to provide definitive mechanistic insights to key questions in the field. We use advanced tools to understand tumor immunity and immunotherapy response. We have developed multiple algorithms to analyze high throughput sequencing data, including RNA-seq, WES, single cell, spatial transcriptomics, ATAC-seq data, etc. When data lead us to unexpected but interesting results, we also design experiments on the bench hoping to understand the underlying mechanisms. In addition, we have developed multiple experimental platforms such as CRISPR screens, mouse models, cellular immunology, and molecular biology to perturb both immune and cancer cells and study their effects in the tumor microenvironment. These approaches will enable a new understanding of the tumor microenvironment and provide new ideas for clinical immunotherapy.
CRISPR techniques
Our research focuses on employing CRISPR techniques to identify and validate functional gene targets in immune cells, such as CD8+ T cells, CD4+ T cells, NK cells, macrophages, and B cells. By collecting and integrating a large volume of genome-wide CRISPR screen data, designing CRISPR screen experiments, and developing machine learning models, we aim to uncover key gene regulators involved in cancer immunology and immunotherapy response. Additionally, we are working on combining spatial techniques with CRISPR technology to better understand immune cell interactions within the tumor microenvironment. Promising targets identified through our models will be validated using high-throughput experimental platforms for further investigation and potential therapeutic development.
Spatial transcriptomics
We focus on advanced nanoscale (or subcellular-level) spatial transcriptomics technology. By combining image segmentation algorithms based on deep generative models to reconstruct spatial single-cell transcriptome, we use transcriptome data to fine-tune and optimize cell boundaries, thereby developing a set of algorithms and pipelines for data processing, analysis, and mining. These include the identification and inference of cell-cell interaction, cell differentiation trajectory, RNA velocity and spatially variable genes, the extraction of spatial feature regions, and the evaluation of tumor immune infiltration using spatial single-cell transcriptome and deep learning models. In addition, we collaborate with clinical doctors to sequence tumor samples using nanoscale spatial transcriptomics and other multi-omics technologies to generate spatial transcriptomics datasets, and draw the spatial transcriptomic atlas for multiple cancer types. We are also developing nanoscale spatial transcriptome technologies for immune repertoire and CRISPR screening, and corresponding analysis tools. We will establish multi-modal data mining models of spatial transcriptomics, proteomics and epigenomics to further decipher the tissue heterogeneity and tumor microenvironment of primary and secondary tumors.
Single cell and other omics
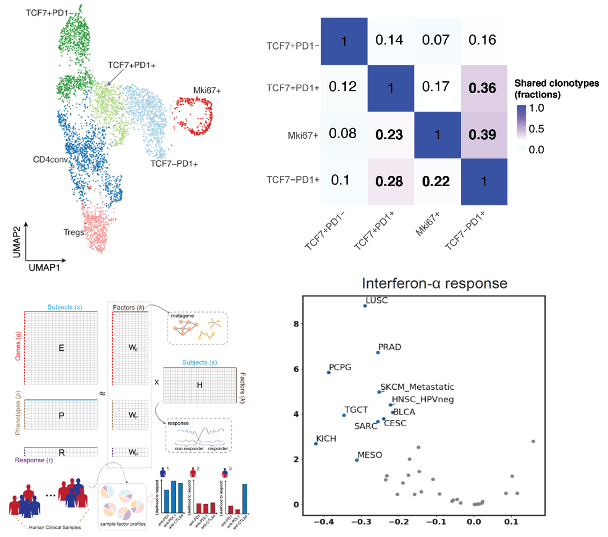
We are using omics data to investigate the complex factors influencing antitumor immunity in single-cell research. To handle the growing data complexity, we're developing machine learning and statistical methods. By analyzing these data, we aim to better understand the interplay between different biological factors in the tumor microenvironment and identify key regulatory genes. Our research will help unravel the regulatory networks within the tumor microenvironment and shed light on previously underappreciated biological factors in antitumor immunity, paving the way for more effective cancer treatments.