The World Health Organization speculates that by 2050, deaths caused by antibiotic and anti-cancer drug resistance will become the biggest killer of humans. In clinical practice, the emergence of acquired resistance mutations of pathogens or cancer cells is almost inevitable. After a period of use, the drug will become inefficient or ineffective. For example, EGFR kinase targeted drugs are currently the first-line treatment for patients with non-small cell lung cancer, which significantly improves the treatment effect and quality of life of patients. However, because tumors evolve drug resistance, making the first round of drugs used for treatment ineffective. Drug research and development personnel can only continue to develop a new generation of drugs to combat individuals with drug-resistant mutations, which generally requires a large amount of R & D investment and several years, lagging behind the emergence of drug resistance. In March 2017, the third-generation EGFR inhibitor osimertinib was marketed in China. With the widespread use of first- and second-line osimertinib, the problem of resistance to osimertinib has become increasingly apparent. It has just entered Phase I clinical trials, and some patients are currently facing a situation where no drugs are available.
In order to speed up the development of anti-drug resistance drugs, the team of Professor Lai Luhua and Professor Pei Jianfeng developed a new strategy that can predict drug resistance mutations in advance. Prediction and discovery, based on this strategy, it is expected to be able to develop a new generation of anti-drug mutant drugs 1-3 years in advance, which provides new ideas for solving drug resistance problems. Their strategy includes a combination of calculations and experiments. The calculation part (EVER algorithm) simulates the evolution process of mutants, including three main steps: DNA sequence evolution of drug binding sites, protein structure modeling and docking, and construction and selection of scoring system. Using EGFR kinase targeting drugs (gefitinib) and BCR-ABL kinase targeting drugs (imatinib, nilotinib, and punitinib) EVER successfully predicted a variety of drug resistance mutations that have been observed in the clinic. EVER not only considered the kinase-drug combination, but also that the mutation could not destroy or significantly increase the enzyme activity.
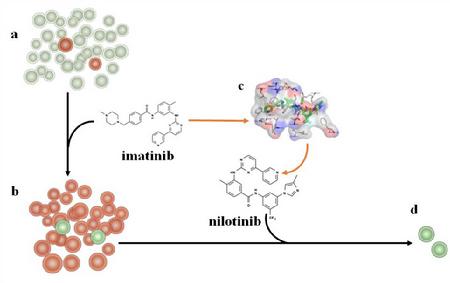
Figure 1. Schematic diagram of a new strategy for predicting drug resistance mutations. Most of the initial cancer cell population (a) are drug-sensitive cells and a small number are drug-resistant cells. After a period of clinical treatment with the first-generation drugs, the drug fails and the cancer cell population gradually changes to the state (b); The second-generation drugs must be used for treatment to continue killing cancer cells; after a period of application, the second-generation drugs are ineffective, and the third-generation drugs are required. This process is repeated, and the development of a new generation of drugs needs to keep up with the development of titanium sulfate; and patients who are resistant to the latest generation of marketed drugs face the dilemma of no drug availability. The new strategy for the development of anti-drug drugs developed in this work (c) allows the prospective research and development of next-generation drugs can be carried out in the first 1-3 years before the emergence of drug-resistant cells, thereby accelerating the development of drug-resistant drugs and preparing drug reserves for clinical drug-resistant mutations.
In the in vitro experimental verification of the calculated prediction results, it was further found that the predicted mutation types can cause a certain degree of reduction in binding capacity, but half of the inhibitory concentration does not necessarily change in the same proportion. Drug-resistant mutations that occur in clinical settings generally do not significantly change the enzyme kinetic constants, and the enzyme activities of mutants that do not appear in the clinic often change significantly, indicating that the kinase activity is strictly regulated by the organism; Only when the inhibitory concentration exceeds a certain threshold will clinical resistance occur. Based on the above results, they proposed a new strategy for effectively predicting resistance mutations of kinase drugs: first use the EVER algorithm to predict possible drug-resistant mutants, and then use in vitro experiments to determine the drug's half-inhibitory concentration of the predicted mutants, if half If the change of the inhibitory concentration exceeds the safety window of the drug, the mutant is likely to appear in future clinical practice. Through this strategy, a new generation of drugs can be designed in advance for potential drug resistance mutations.
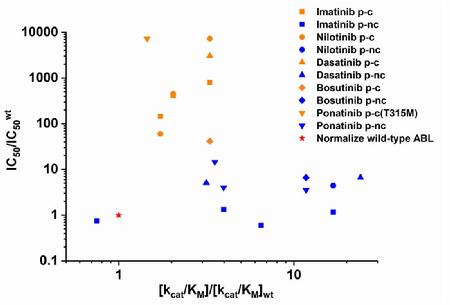
Figure 2. Compared with the wild type, the drug has a higher half-inhibitory concentration of drug-resistant mutants that occur in the clinic. Drug-resistant mutants that occur in the clinic usually do not significantly change their enzyme kinetic constants; and the half-inhibitory concentration of the drug for the mutants that did not appear in the clinic did not change much, and the enzyme kinetic constants of the mutants that did not appear in the clinic generally changed more than the wild-type. Drug-resistant mutants that occur in the clinic and those that do not appear in the clinic have a clear separation interval.
The research was published in the Journal of Communications Biology (https://www.nature.com/articles/s42003-019-0743-5). The 2014 PhD student Liu Jinxin who has graduated from the PTN project is the first author of this article, and Professor Lai Luhua and Professor Pei Jianfeng are the corresponding authors of this article. The thesis work was assisted by Dr. Zhang Weilin, Dr. Xu Youjun, and CLS 2019 doctoral student Deng Bo and others. This work has received funding and support from the National Natural Science Foundation of China and the National Key R & D Program.