北京大学定量生物学中心
学术报告
题 目: Graph Neural Networks to Learn Long-range Interactions in Proteins from Molecular Dynamics Simulations
报告人: Professor Dong Xu
Department of Electrical Engineering and Computer Science, Bond Life Sciences Center, University of Missouri, Columbia, MO, United States
时 间: 1月11日(周一)9:00-10:00
地 点: Online (Zoom会议)
会议号:627 9242 4126
密码:cqbcqb
主持人: 宋晨 研究员
摘 要:
Protein allostery is a spatially long-ranged regulation, whereby ligand binding or amino acid mutation at distant sites affect the active site remotely. Atomic/residue interactions often drive such biomolecular motion related to protein function. Molecular dynamics (MD) simulation can probe the biomolecular motion, but it is still challenging to develop the MD analysis method to capture meaningful functional information from the high dimensional and complex 3D data. In this study, we applied a neural relational inference (NRI) model based on a graph neural network (GNN) to analyze MD simulations in biological systems. The NRI model consisting of an encoder and a decoder, which can simultaneously infer latent interactions while reconstructing the dynamic trajectories. This model allows us to formulate the protein allosteric and activation processes as the dynamic networks of interacting residues. In the allosteric regulation case studies, protein Pin1 utilizes the allostery induced by ligand binding to regulate its function. NRI model successfully learned the pathways mediating the allosteric communication between the two distant binding sites. Furthermore, for the SOD1 and MEK1 systems, the NRI model also learns the interaction between domains/residues, driving the protein’s slow-motion regulated by mutations at distant sites. Our work provides a practical analysis method for probing structural correlations related to function.
报告人简介:
Dong Xu is Professor in the Electrical Engineering and Computer Science Department with appointments in the Christopher S. Bond Life Sciences Center and the Informatics Institute at the University of Missouri-Columbia. He was awarded the Paul K. and Dianne Shumaker Endowment in Bioinformatics in 2018. He obtained his PhD from the University of Illinois, Urbana-Champaign in 1995 and did two years of postdoctoral work at the US National Cancer Institute. He was a Staff Scientist at Oak Ridge National Laboratory until 2003 before joining the University of Missouri, where he served as Department Chair of Computer Science during 2007-2016. His research is in computational biology and bioinformatics, including machine-learning application in bioinformatics, protein structure prediction, post-translational modification prediction, high-throughput biological data analyses, in silico studies of plants, microbes and cancers, biological information systems, and mobile App development for healthcare. He has published nearly 300 papers. He was elected to the rank of American Association for the Advancement of Science (AAAS) Fellow in 2015 and American Institute for Medical and Biological Engineering (AIMBE) Fellow in 2020.
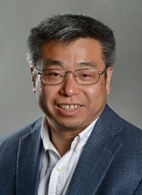